Countries
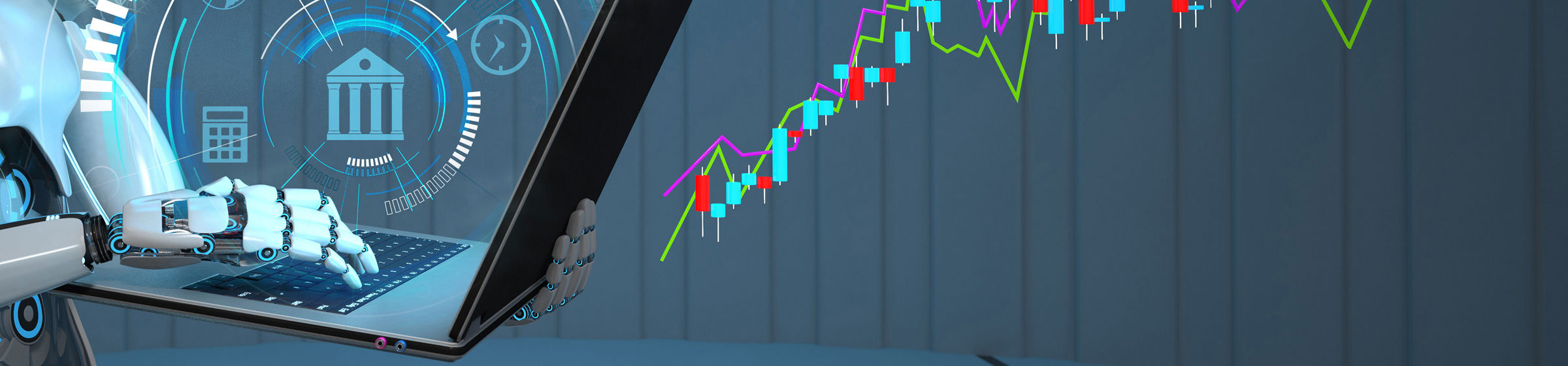
Financial algorithmic trading: Humans and machines join forces
In financial algorithmic trading, expectations of future trends in the energy market are bought and sold based on automated trading patterns. The interaction between machines and humans opens up completely new possibilities.
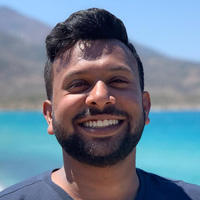
Laxman Pararasasingam is a trader in Options and Systematic Trading, in the Markets business area of Statkraft.
Statkraft is a key player in the market for futures contracts in energy. Futures contracts are agreements to buy or sell a commodity in the future at a certain price. The commodity could be power, gas, oil, carbon emissions, coal and more.
“Buyers of futures contracts often try to reduce the risk that prices will go up, while sellers try to hedge against them falling. Regardless of the actual outcome, it helps both buyers and sellers reduce risk and uncertainty by locking in a price that is acceptable in their future income streams,” explains Laxman Pararasasingam, who is a trader in Statkraft's options and systematic energy trading unit in the business area Market and IT.
Commercial opportunities
Participants in the futures market are comprised of hedgers and speculators. Hedgers carry out trades not specifically to make a profit, but to minimise exposure to market risk, while speculators look to profit from the fluctuations by taking over the aforementioned risk.
“An owner of a producing asset may want to secure the future value of its production by selling futures (hedging) to lock in the income for the remaining life of the asset. The intention is to 'buy insurance' and reduce future uncertainty instead of trying to predict where prices will go. This gives rise to an insurance premium or risk premium,” says Pararasasingam.
For a company like Statkraft, which has a large market risk exposure and considerable expertise in the energy markets, this opens up many commercial opportunities.
“Some departments act as a 'market risk aggregator' for smaller consumers and producers, other departments are responsible for hedging our own assets. Whereas my team is engaged in proprietary trading. The goal is to profit from accumulating the aforementioned risk and take an active approach to risk management. One of the ways we mitigate this risk is by diversifying into other commodities that affect the power market, such as gas, coal, oil or carbon emissions,” he says.
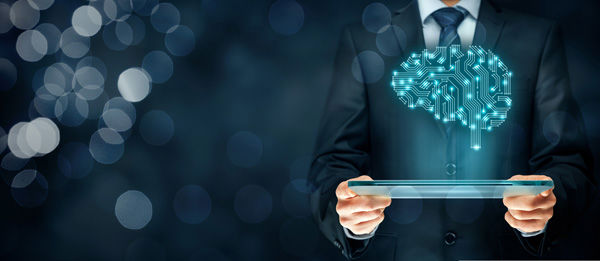
Algorithmic trading in the financial markets
Algorithms can calculate trends in the financial energy market in a completely different way than what humans can achieve alone. Machines process large amounts of data in an instant, so that decisions based on historical patterns can be more reliable.
Trading algorithms come in several variations:
- The decision to place an order is generated by an algorithm and executed by a human.
- The decision to make a trade is taken by a human and executed by an algorithm.
- An algorithm decides to make a trade, which is then executed in a fully automatic way – with human supervision, but not necessarily human intervention.
The basic drivers of financial algorithm trading are long-term expectations of demand and supply, geopolitical events and changes in policies and regulations.
“The time horizon can be anything from weeks or months to five years ahead,” says Pararasasingam.
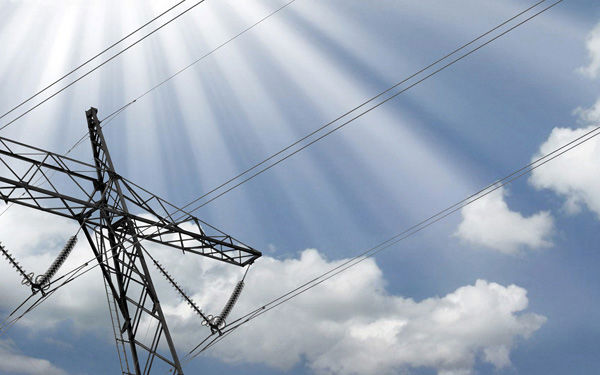
Robust framework
Large sums and high risk can be involved in financial algorithm trading.
“As a result, trading based on financial algorithms is strictly regulated. Many of the regulations in energy trading are based on similar rules from the financial sector and are not necessarily directly transferable. This particularly applies to illiquid energy markets. When the regulations are changed to deal with this difference, we must constantly learn to take the changes into account. We have heard horror stories about algorithms crashing the financial market, so it's crucial to have a robust framework for financial algorithm trading,” says Pararasasingam.
In Statkraft's financial algorithm trading unit, algorithms handle the calculations, and then the team makes decisions based on the results.
“The algorithms give us much greater flexibility, because they allow us to scale up and react quickly in different products and markets. The most valuable approach for us is to use algorithms to process data and then leave it to humans to make the decisions. Our ambition is not to be the fastest, but the smartest player in the energy market,” he adds.
Pararasasingam believes algorithms will play an increasingly important role in the future.
“The volume of data to be processed will only increase, and therefore the expertise to handle this must be strengthened and expanded in a sound manner,” says Laxman Pararasasingam.
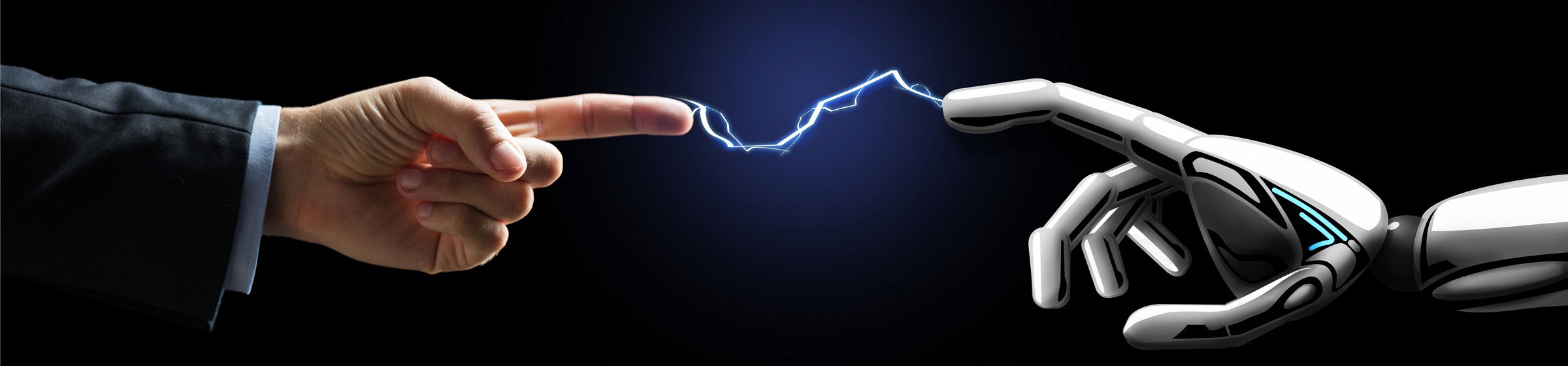
See also: Physical algorithmic trading
With an ever-increasing share of renewable energy sources in the power grid, digital solutions for energy trading have become indispensable. Based on carefully developed algorithms, traders can optimise power generation and power deliveries in real time.
Physical algorithmic trading: Quick decisions